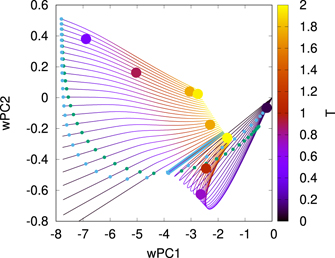
Our article on using unsupervised machine learning to analyse neutron scattering functions is now published.
In this work we deploy very similar tools to those used in another recent paper on muon spin relaxation measurements, except that in this case we consider theoretically the potential such techniques have to interpret neutron scattering measurements. As outlined in a recent talk this way of doing condensed matter physics where an algorithm capable of unsupervised learning (in this case, Principal Component Analysis, or PCA) is used to interpret observable properties of condensed matter systems can be described as a form of “augmented intelligence”: the machine carries out tasks that were previously executed by humans, such as spotting trends in the data, identifying qualitative changes, and finding a compact description of the observed phenomenology, leaving the investigator to focus on other aspects of the problem.
The image accompanying this post, which can be found in our recent paper, shows a geometric construction that can be used to obtain, using finite-temperature data, the field at which a qualitative change in the phase diagram of a spin system takes place. Interestingly, in earlier work investigating the so-called “entanglement transition” in such systems we found that this change in the system’s ground state shows up in observables as a smooth crossover at any finite temperature, so the fact that our PCA can be used to obtain the critical field value from data obtained at finite temperature is quite remarkable.
The reference and abstract are given below. This is published under Gold Open Access so follow the link straight to the publisher to get the most up to date version.
- Robert Twyman, Stuart J. Gibson, James Molony, and Jorge Quintanilla
“Principal Component Analysis of Diffuse Magnetic Neutron Scattering: a Theoretical Study”
Journal of Physics: Condensed Matter, 33 (32), 324002 (2021)
DOI:10.1088/1361-648X/ac056fWe present a theoretical study of the potential of principal component (PC) analysis to analyse magnetic diffuse neutron scattering data on quantum materials. To address this question, we simulate the scattering function S(q) for a model describing a cluster magnet with anisotropic spin–spin interactions under different conditions of applied field and temperature. We find high dimensionality reduction and that the algorithm can be trained with surprisingly small numbers of simulated observations. Subsequently, observations can be projected onto the reduced-dimensionality space defined by the learnt PCs. Constant-field temperature scans correspond to trajectories in this space which show characteristic bifurcations at the critical fields corresponding to ground-state phase boundaries. Such plots allow the ground-state phase diagram to be accurately determined from finite-temperature measurements.