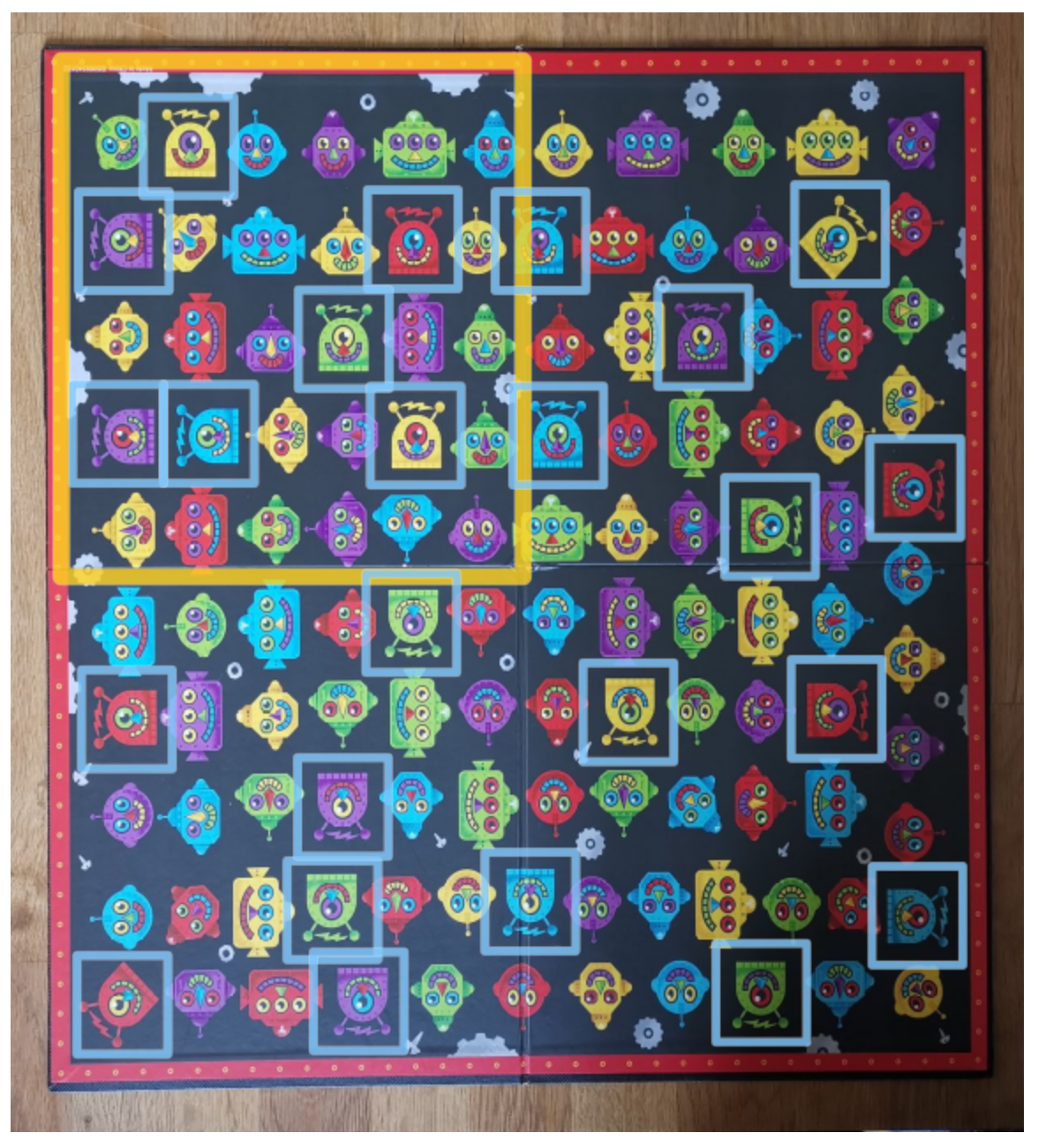
Talk on Machine Learning given at "Condensed Matter and Quantum Materials (CMQM 2021)".
Today I gave two talks at “Condensed Matter and Quantum Materials (CMQM 2021)”. Here’s the abstract of one of these talks. The image shown is from one of the slides I used, where I explain the differences between supervised an unsupervised learning using the RobotFace gameboard as an example (that was fun – for me at least!) Follow the link to view/download all the slides using SlideShare.
Sessions:
SCES / Quantum Magnets / Superconductivity
Title:
“Principal Component Analysis of Quantum Materials Data: a Study in Augmented Intelligence”
Authors:
D S Barker (1,5), S R Giblin (2), A D Hillier (3), E E McCabe (1,4), G Möller (1), James Molony (1), J Quintanilla (1), S Ramos (1), T Tula (1), Robert Twyman (1,6)
(1) University of Kent (2) University of Cardiff (3) ISIS Facility (4) University of Durham (6) UCL
Abstract:
There is much interest currently in the potential of machine learning to tease useful information out of complex data on materials. Here we ask whether this can work when only experimentally accessible data, i.e. averages rather than microstates, are available. We use Principal Component Analysis to study simulated neutron-scattering data on cluster quantum magnets [1] and experimental muon-spin relaxation curves from various superconducting and magnetic materials [2]. While the algorithms can perform certain functions, such as detection of phase transitions, automatically, I will argue that their best use is in providing human scientists with new ways to look at the data – an approach that can be best characterised as “augmented”, rather than “artificial”, intelligence.
References:
[1] R. Twyman, S. J. Gibson, J. Molony, J. Quintanilla, “Principal Component Analysis of Diffuse Magnetic Scattering: a Theoretical Study”, Special Issue on Machine Learning in Condensed Matter Physics, J. Phys.: Condens. Matt. (accepted). arXiv: 2011.08234.
[2] T. Tula, G. Möller, J. Quintanilla, S. R. Giblin, A. D. Hillier, E. E. McCabe, S. Ramos, D. S. Barker, S. Gibson, “Machine Learning approach to muon spectroscopy analysis”, Special Issue on Machine Learning in Condensed Matter Physics, J. Phys.: Condens. Matt. 33, 194002 (2021). DOI: 10.1088/1361-648X/abe39e.